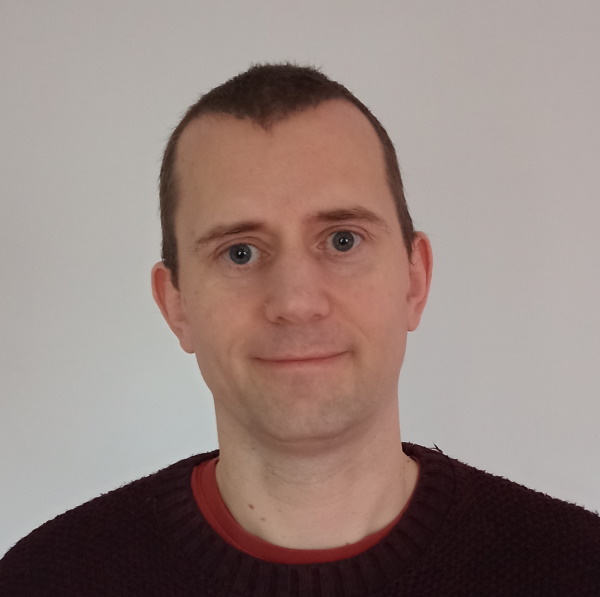
Mark is a coding architect specializing in building microservices with .NET on Azure. He also enjoys writing audio-related code and created the NAudio framework. He has created over 20 courses on Pluralsight, and would love to speak at your conference or user group event.
Recent posts
- 3 Apr 2024 Configure Azure Functions to use Identity Based Connections
- 8 Jan 2024 Some Stream.Read Guidelines
- 1 Jan 2024 2023 in review
- 26 Dec 2023 What are we optimizing for?
- 24 Nov 2023 Creating Zip Files in Azure Blob Storage
- 31 Aug 2023 Playing MIDI through SoundFonts with NAudio and MeltySynth
- 22 Aug 2023 Cleaning up dangling DNS entries for an Azure DNS Zone
- 17 Aug 2023 Using ETags and Patching Rows in Azure Table Storage
- 27 Jul 2023 Stream videos from Azure Blob storage with ASP.NET Core
- 30 Jun 2023 Pass variables between steps in Azure Pipelines